Mẹo về Tend to add value that is greater than the cost to incorporate them into the supply chain. 2022
Lê Bình Nguyên đang tìm kiếm từ khóa Tend to add value that is greater than the cost to incorporate them into the supply chain. được Update vào lúc : 2022-10-22 03:46:17 . Với phương châm chia sẻ Kinh Nghiệm Hướng dẫn trong nội dung bài viết một cách Chi Tiết 2022. Nếu sau khi tham khảo Post vẫn ko hiểu thì hoàn toàn có thể lại phản hồi ở cuối bài để Tác giả lý giải và hướng dẫn lại nha.1. Grocery Retail Strategies Fall Flat If Not Backed by the Right Supply Chains
Food retail is a tough and turbulent market. Grocery has never been easy, but the current business transformation is more dramatic than anything we have seen in decades, especially with COVID-19 further accelerating many trends shaping the market.
Nội dung chính- 1. Grocery Retail Strategies Fall Flat If Not Backed by the Right Supply Chains2. Harness the Power Of AI to Optimize Your Grocery Supply Chain3. Demand Forecasting is the Engine Running Your Grocery Supply Chain3.1. Granular, Data-Driven Forecasting Is A Must for Grocery Retailers 3.2. Machine Learning Delivers High Value In Grocery Demand Forecasting 3.3. Typical Demand Forecasting Challenges for Supermarkets, Discounters, and Convenience Stores 3.3.1. Predicting Demand for New Products and Stores3.3.2. Forecasting The Impact Of Promotions And Price & Display Changes3.3.3. Considering Cannibalization and Halo Effects in Forecasting3.3.4. Estimating The Impact of Weather and Other External Factors on Demand3.3.5. Dealing with Unexplained Changes in Demand3.3.6. Incorporating Planner Expertise In Demand Forecasting4. Refine Grocery Replenishment for Improved Availability, Waste, and Efficiency4.1. Fresh Food Replenishment Requires Detailed Planning and Execution4.1.1. Balancing Waste and Lost Sales4.1.2. Stores Have Turned into Kitchens4.1.3. High-frequency Replenishment for Ultra-Fresh Products4.1.4. Adding Science to the Art of Managing Fruits and Vegetables 4.2. Optimized Center Store Replenishment Is Key to Supply Chain Efficiency4.2.1. Synching Replenishment and Shelf Space for Cost-Efficient Operations4.2.2. Smart Replenishment For Efficient Store Operations And More Level Goods Flows4.2.3. Dynamic Pack Sizes to Meet Dynamic Demand5. An Integrated Supply Chain Driven by Customer Demand5.1. Plan Once and Execute Automatically Throughout the Supply Chain5.2. Multi-echelon Optimization of Goods Flows6. Efficient Inventory Management in Distribution Centers6.1. Total Cost Optimization of Inbound Flows6.2. Smart Buying Takes Advantage of Good Prices6.3. Batch-Level Inventory Management of Perishables6.4. Real-time Data for Buying Fresh Products7. Planning for Optimal Capacity and Resource Utilization Throughout the Grocery Supply Chain7.1. Retail Sales & Operations Execution (S&OE)7.2. Retail Sales & Operations Planning (S&OP)7.3. Efficient Supplier Collaboration 8. Conclusion: Team up with the Machines to WinWhat tend to add value that is greater than the cost to incorporate them into the supply chain?What are the 4 types of customer value?What is Consumer market value?What is the key to creating value for consumers?
Grocery retailers need to simultaneously address several major trends:
- Breakneck developments in online ordering and
order fulfillment options. From online ordering to home delivery and curbside pickup, the speed of omnichannel development has made operational efficiency particularly challenging for food retailers due to their mix of low-value products and high handling costs for fragile, variously sized products that often require temperature control. But while many struggle to make e-grocery profitable, few food retailers can afford not to go online.Discounters have demonstrated the
power of operational efficiency. Since 2008’s financial crisis, discounters have grown their market share, proving that shoppers appreciate low-cost private label products and well-curated assortments, even the expense of abundant choice. Cost control always provides a competitive advantage, especially when consumers are hesitant to spend, so streamlining operations to improve efficiency must be a key part of every grocery retailer’s strategy going forward.Grocery
retailers must also compete with the food service industry. Restaurants, meal delivery services, and food-to-go are capturing an increasing share of consumers’ wallets. As a result, food retailers are turning to prepared meals to increase relevance and high-value food-to-go items to improve margins in a tough environment. Some are even opening in-store restaurants. With poor execution, though, these initiatives also introduce new opportunities to lose money on costly food waste.
Spotlight on challenging fresh and short shelf life products. Healthy eating trends have even discounters like Aldi and Lidl improving their fresh offerings to include organic meats and freshly baked breads. In their pursuit of growth, these former hard discounters are stepping away from their highly efficient comfort zones previously built on simplicity, standardization, and large volumes. The increasing complexity as they experiment with fresher products, smaller store
formats, and localized assortments will put their grocery supply chains to the test.The push toward sustainability. Consumers’ environmental concerns are only growing, and they expect their grocers to develop sustainable practices too. Many leading retailers have already committed to lowering their carbon emissions, but very soon, sustainability will move from “positive messaging” to table stakes. Retailers who fail to take meaningful action to reduce waste and
emissions put their reputations risk. The good news is that sustainability, efficiency, and profitability, in many cases, go hand in hand.
These trends all present challenges and opportunities, but it’s clear that supply chain management will lie the heart of the successes—and failures—we see in the future of grocery retail. All food retailers need to make tough choices today about where to place their business bets. Whichever strategy a retailer chooses, they have a slim chance of success if they don’t develop their grocery supply chain to match it.
Successful food retailers need to master both the hard discounters’ lean, highly efficient grocery supply chains as well as the agile, responsive supply chains needed for fresh products. In addition, many of them will need to manage the complexity of operating multiple store formats while offering several fulfillment options.

To achieve this, retailers need the right planning tools their disposal. Furthermore, they need to understand how to apply them.
- Fresh products typically have a high risk of markdowns and waste, making it very important to accurately forecast
demand and replenish in sync with it. For fresh products, the planning process must be granular enough to capture even the smallest changes in demand and the supply chain agile enough to adapt to variable demand.Center store and other longer shelf life products are key to efficient goods handling and inventory flow optimization. Though accurate forecasting remains essential, replenishment for long shelf life products does not need to be exactly in sync with demand
any given moment, making it possible to level out the inventory flow through the supply chain for efficient capacity utilization. Setting up store deliveries to allow for one-touch replenishment or “truck-to-shelf” delivery is essential for increased profitability.Omnichannel has grown to a point where it can no longer be operated as an “add-on” service exempt from standard efficiency requirements. Online fulfillment actually accentuates the need for high quality and
freshness because end-consumers can’t evaluate products for themselves. Furthermore, with many grocers today taking significant losses on online orders, the continued growth of omnichannel highlights the need for significant improvements to operational efficiency.
In this best practice guide, we will highlight key approaches for increasing both responsiveness and efficiency in grocery supply chains. You will be hard pressed to find a single retailer employing all of these best practices. Rather, we encourage you to prioritize the most feasible and impactful development areas from your own perspective.
2. Harness the Power Of AI to Optimize Your Grocery Supply Chain
Today’s grocers collect massive amounts of data on transactions and interactions with consumers both on- and offline. That’s precisely why grocery retail is the perfect match for artificial intelligence (AI), which makes it possible to leverage that data into faster, more accurate decisions. This is an invaluable asset in an industry where retailers must control millions of goods flows and accurately match supply to demand hundreds or even thousands of locations on a daily basis.
Technology companies can be eager to position their AI algorithms as “intelligent” by making them as human as possible—even giving them human names like Siri, Alexa, Einstein, or Watson. Keep in mind, though, that AI is not a person. AI is not even a singular “it.”
We are still far from general artificial intelligence that would be able to creatively solve ill-defined problems. We are, however, making great progress in specialized AI that solves well-defined problems (such as algorithms for image recognition) and combinations of several types of specialized AI (such as self-driving cars).

Figure 2: Which is the better robot for washing clothes? Specialized AI is growing increasingly common and is often used to run applications that, first sight, do not look particularly intelligent. (Illustration inspired by an excellent blog post by Ben Evans.)
Retailers do not need “AI”—they need to employ several AI algorithms in their analytics toolbox to supplement “old hat” technologies like statistical analysis and rules-based heuristics. Machine learning algorithms, for example, consider hundreds of potentially demand-influencing factors when forecasting retail sales (Section 3.2), something a human demand planner could never achieve.
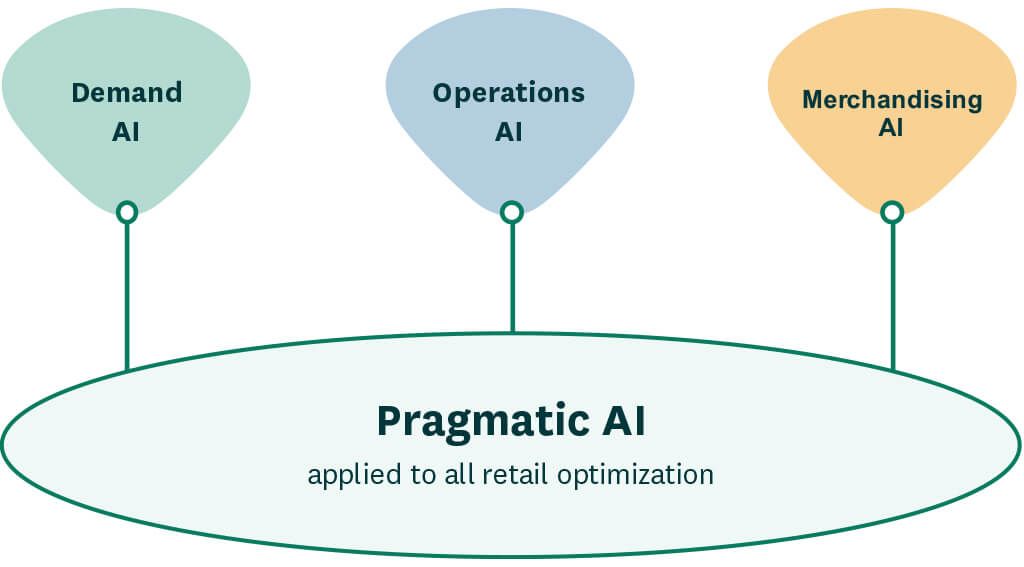
The application of AI in grocery retail is not limited to demand forecasting, though. Retailers can reap even greater benefits by leveraging AI to optimize the full range of their operations – from order optimization (see section 4.1.1) to workforce optimization (read the whitepaper) and optimized markdowns (read the whitepaper).
3. Demand Forecasting is the Engine Running Your Grocery Supply Chain
Demand forecasting is the engine running your grocery supply chain. Yet, despite the technology available, a great number of notable grocery retailers have yet to truly embark on their journey of data-driven forecasting.
3.1. Granular, Data-Driven Forecasting Is A Must for Grocery Retailers
In a 2022 survey of North American grocery retailers, only 52% of respondents reported that they could produce forecasts on the day-SKU-store level (SKU = stock-keeping unit). The rest wanted to do day-level forecasting, but simply weren’t able to. Few retailers today are able to forecast online orders picked in their stores separately either, which inevitably leads to capacity management issues, especially after the significant growth of online demand fueled by the COVID-19 crisis.
Granular forecasting is not just a best practice—it’s a must-have in today’s grocery retail. Without detailed forecasts, it’s impossible to correctly position inventory in the supply chain to maximize sales and minimize waste. Granular forecasts are also the planning foundation for both resource and capacity management, and thus should be considered a prerequisite for profitable operations.
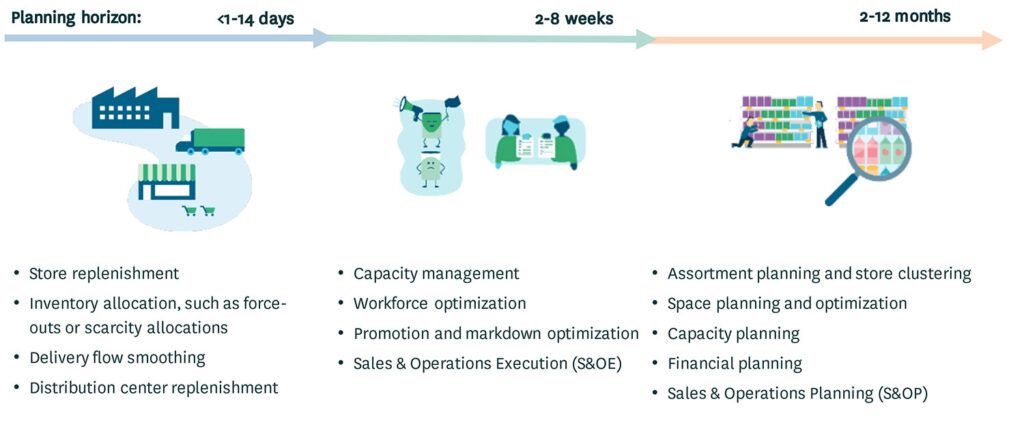
3.2. Machine Learning Delivers High Value In Grocery Demand Forecasting
What started with a few forerunners like RELEX has become mainstream over the years: machine learning for retail demand forecasting. Currently, retail technology vendors either apply machine learning or are rushing to update their legacy systems to offer it.
Machine learning gives a forecasting system the ability to learn automatically and improve its predictions using data alone, with no additional programming needed. Because retailers generate enormous amounts of data, machine learning technology quickly proves its value.
Of course, machine learning algorithms are not new—they’ve been around for decades. But never before have they been able to access as much data or data-processing power as is available today. Though grocery retailers may have struggled to update their forecasts quickly in the past, large-scale data processing and in-memory technology now enable millions of forecast calculations within the space of a single minute.
Machine learning makes it possible to incorporate a wide range of demand-influencing factors and relationships into your grocery sales forecasts. This is enormously valuable, as weather data alone can consist of hundreds of different factors that can potentially impact demand.
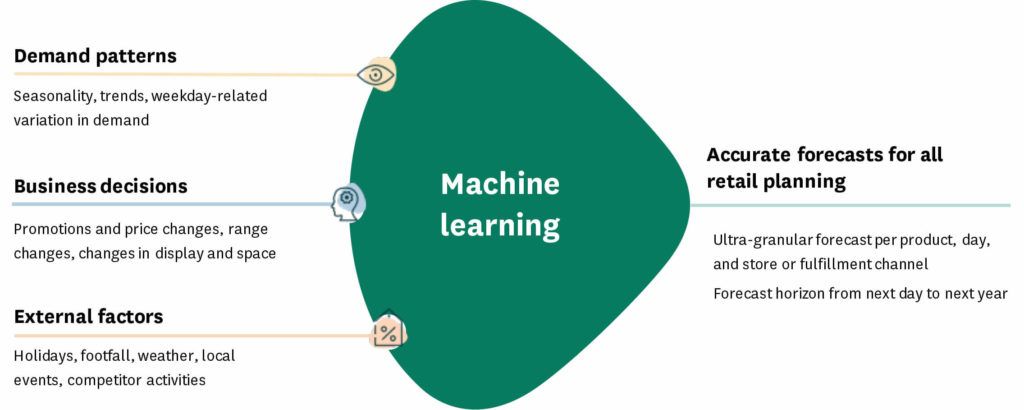
However, a word of caution is called for here: it really does make a difference how machine learning is implemented. Although grocery retailers are able to collect massive amounts of data, their data is often quite limited on the store and SKU level. Slow-moving products may not provide enough sales transactions to study; master data on past promotions and product displays may be lacking; and as products move in and out of range, data may not be able for the exact SKU they’re attempting to forecast. Machine learning forecasting must be set up correctly to make it less vulnerable to data issues, which would cause a “garbage in, garbage out” scenario.
Implemented the right way, machine learning effectively addresses common challenges with retail data to deliver benefits across all facets of grocery demand forecasting: 1) capturing recurring demand patterns caused by weekdays and seasons, 2) forecasting the impact of promotions, price changes, and other internal business decisions, 3) predicting the impact of local footfall, events, weather, and other external factors, and 4) even detecting when unknown factors (such as an unrecorded change in how a product is displayed) may be impacting demand.
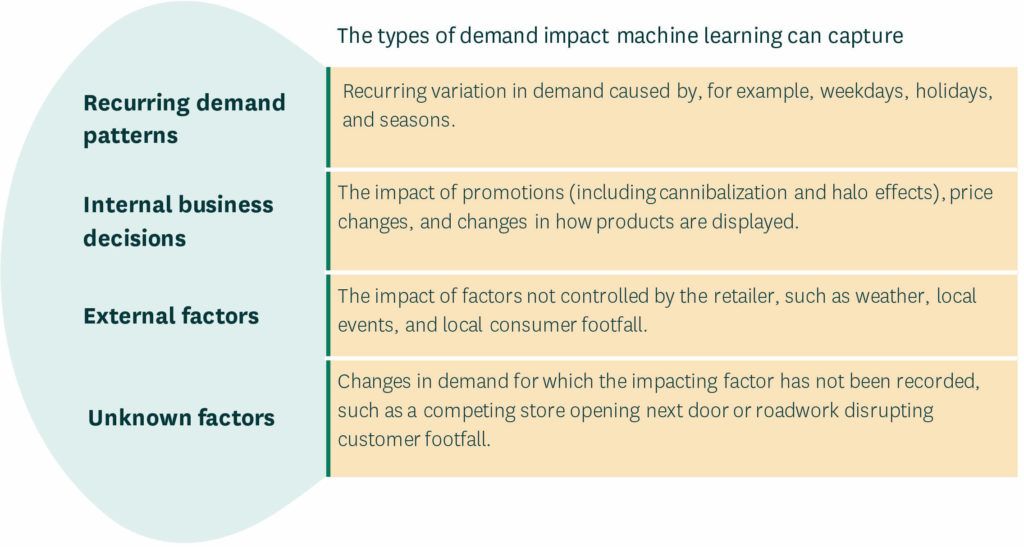
In simple scenarios like forecasting a predictably recurring demand variation, machine learning delivers only equivalent or slightly better accuracy than traditional time series-based demand forecasting. When dealing with complex situations, though, such as overlapping promotions or sales cannibalization, machine learning-based forecasting clearly outperforms traditional forecasting approaches.
3.3. Typical Demand Forecasting Challenges for Supermarkets, Discounters, and Convenience Stores
Next, we will discuss how you can overcome some of the typical forecasting challenges that supermarkets, discounters, and convenience stores face.
3.3.1. Predicting Demand for New Products and Stores
Because machine learning relies on finding patterns in historical sales data, new products with no historical sales data can prove a challenge. Fortunately, further routines are available to improve the management of new product introductions as well.
When introducing a new product, the most common approach is to assign it a reference product to use as a blueprint for its sales pattern until the new product has accumulated sufficient historical data of its own. However, in grocery retail, the number of new products per year can be massive. This makes manually identifying and setting reference products infeasible, or least highly inefficient.
It’s far more efficient to use a system that can automatically compare product attributes (e.g. product group, brand, pack size, color, or price point) to assign the most relevant reference product. The same approach can, of course, be applied to find suitable reference stores for new store openings.

3.3.2. Forecasting The Impact Of Promotions And Price & Display Changes
Your own business decisions as a retailer are also an important source of demand variation, from promotions and price changes to adjustments in how products are displayed throughout your stores. Despite the fact that retailers plan and control these changes themselves, many in the industry remain incapable of accurately predicting their impact.
In the 2022 study of North American grocers, 70% of respondents indicated that they cannot consider all relevant aspects of a promotion—such as price, promotion type, or in-store display—when forecasting promotional uplifts. But they wish they could.
Machine learning allows retailers to accurately model a product’s price elasticity, or how strongly a price change will affect that product’s demand. Price elasticity alone, however, does not capture the full impact of a price change.
A product’s pricing in relation to other products in its category often has a large impact as well. In many categories, the product with the lowest price captures a disproportionally large share of demand. Machine learning-based demand forecasting makes it quite straightforward to consider a product’s price position, as shown in Figure 8 below.
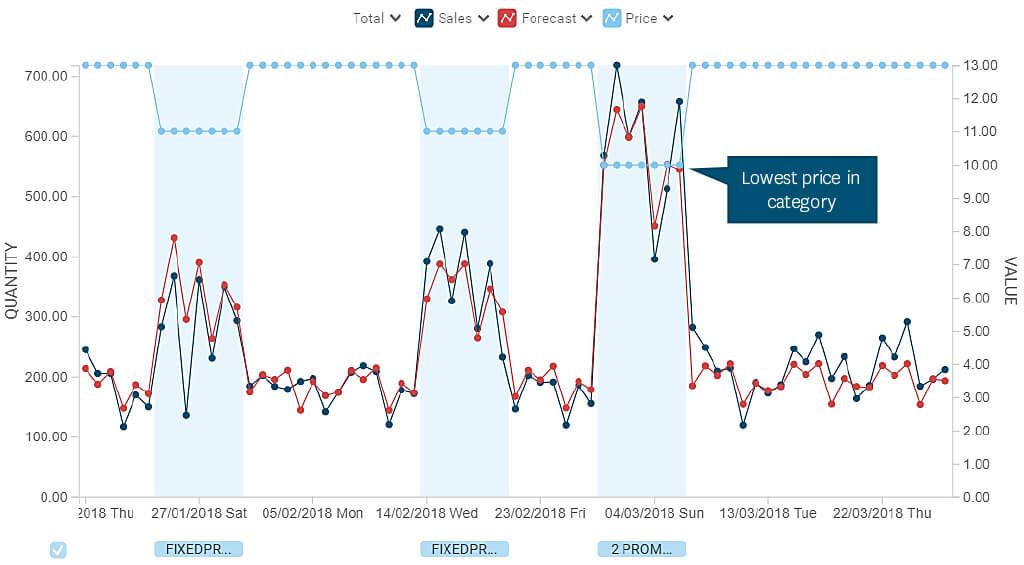
Machine learning does more than simply leverage price data, though. With machine learning forecasting, grocery retailers can accurately predict the impact of promotions by taking into consideration factors including, but by no means limited to:
- Promotion type, such as price reduction or multi-buy Marketing activities, such as circular ads or in-store signage Products’ price reductions In-store display, such as presenting the promoted product in an endcap or on a table
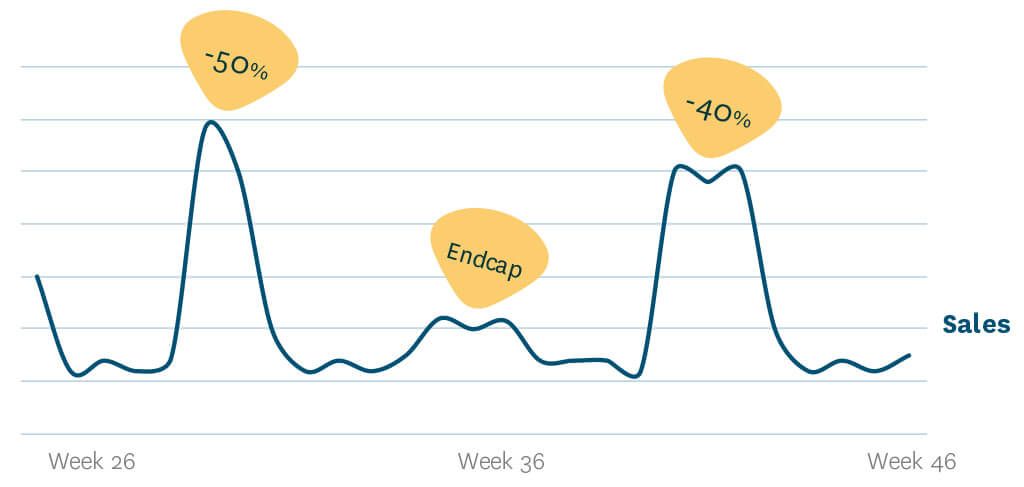
3.3.3. Considering Cannibalization and Halo Effects in Forecasting
It’s quite common for a promotional uplift for one product to actually drive down sales for another. For example, if a supermarket carrying both the “HappyCow” and “GreenBeef” brands of lean organic ground beef puts the HappyCow product on promotion, more people will buy it—but it’s likely that some of the baseline demand for GreenBeef will shift to HappyCow. If they don’t lower the demand forecast for the GreenBeef product, planners are a high risk of stock-piling, leading to waste.
For most center store products, such as canned food or cereal, cannibalization is not a big problem. If demand decreases temporarily, a replenishment order for the cannibalized product will simply be triggered later than usual. However, when working with fresh products and especially products that have a limited number of direct substitutes, forecasts must consider the impact of cannibalization to avoid excess stock and spoilage.
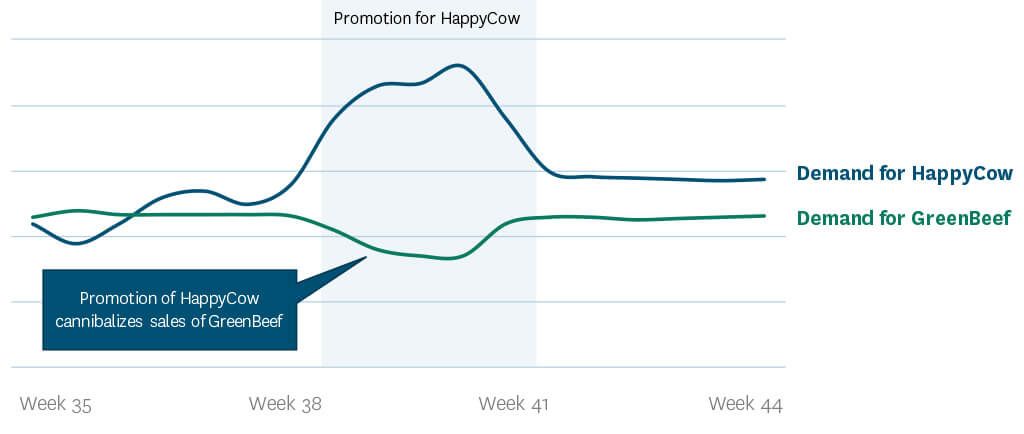
Manually adjusting the forecasts for all potentially cannibalized items is just not feasible in most retail contexts because the number of products to adjust is simply too large. Usually, the patterns are quite specific to individual stores’ assortments and shopping patterns as well. Machine learning algorithms’ ability to automatically identify patterns and adjust forecasts accordingly adds enormous value when addressing sales cannibalization.
The flip side of cannibalization, of course, is the halo effect, or when promoting the HappyCow product also drives sales for related products outside of the “ground beef” class. Hamburger buns, for example, have an obvious and predictable correlation with ground beef.
Unfortunately, the halo effect’s impact can be so diffused across the assortment that identifying every impacted product becomes more or less impossible, even with machine learning. Think onions, potato chips, beer, watermelon, taco meal kits, salad fixings, oyster crackers, corn on the cob, Worcestershire sauce, soy sauce, or any number of other items shoppers might associate with ground beef-based dishes. But even if forecasting systems can’t identify all possible halo relationships, they should still make it easy for planners to adjust forecasts for the relationships they know to exist.
3.3.4. Estimating The Impact of Weather and Other External Factors on Demand
External factors such as the weather, local concerts and games, and competitor price changes can have a significant impact on demand.
It often feels intuitively simple to understand how something like the weather, for example, might impact sales: high temperatures increase ice cream sales, rainfall increases demand for umbrellas, and so on. When looking a retailer’s entire assortment offering, though, it becomes more complicated.
The use of local weather data and forecasts to increase demand forecast accuracy is a great example of machine learning’s power. Machine learning algorithms can automatically detect the relationship between local weather variables and sales for individual products in individual stores.
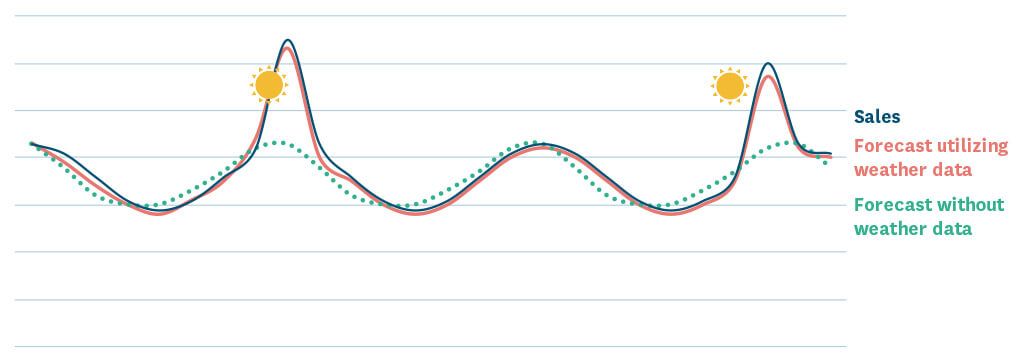
Machine learning algorithms can be used in this same way to take advantage of a wide range of data sources beyond weather alone, helping retailers identify the relationships between external variables such as local sporting events or concerts and local sales for specific products.
In grocery retail, the most useful external data sources include:
- Local weather data and weather forecasts (read the case study) Passenger numbers and forecasts transportation hubs (read the case study) Information on past and future local
events, such as football games or concerts Data on competitor prices
3.3.5. Dealing with Unexplained Changes in Demand
In brick-and-mortar retail, local circumstances—such as a direct competitor opening or closing a nearby store—may cause a change in demand. Unfortunately, data on the factor causing this change may not be recorded in any system. Sometimes, retailers’ own internal decisions also go unrecorded, such as adding a product to a special off-shelf display area in a store.
Fortunately, machine learning can help in these situations. Machine learning algorithms can tentatively place a “change point” in the forecasting model, then track subsequent data to either disprove or validate the hypothesis. This allows forecasts to adapt quickly and automatically to new demand levels.
Consider the example in Figure 12 below, which shows the sales impact when store staff created a table display in addition to the regular shelf space for a product. Though nobody recorded this change in the master data, the system was easily able to track the demand impact as a factor of how the product was displayed in the store.
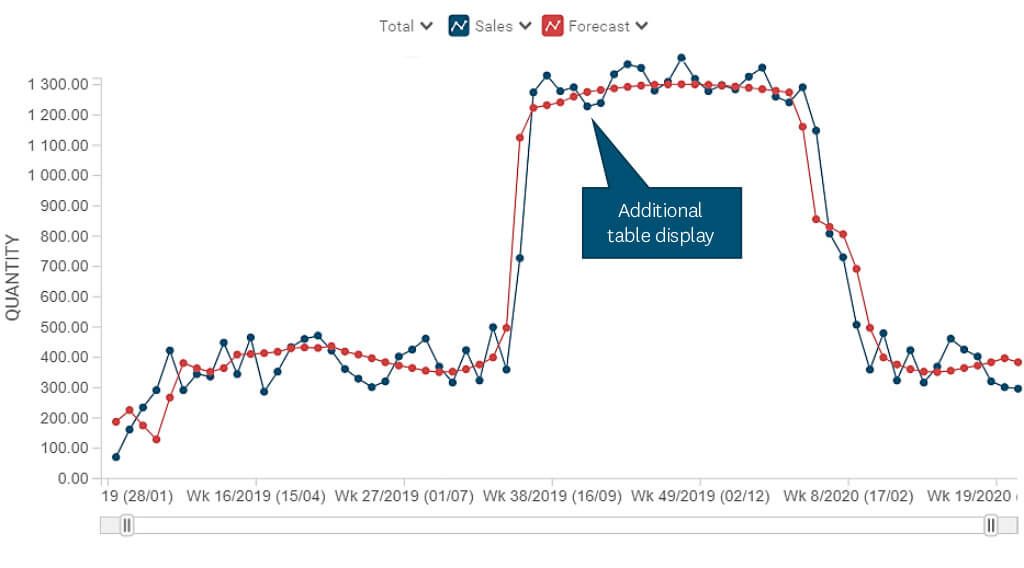
3.3.6. Incorporating Planner Expertise In Demand Forecasting
If you want to remain competitive in food retail, machine learning is something you have to adopt, but you also need to understand its limitations. Automating the majority of your demand forecasting isn’t just desirable—it’s actually quite feasible with machine learning. But the COVID-19 crisis demonstrated clearly that there will always be circumstances in which system-generated forecasts will be off (although some systems can recover more quickly than others).
The pandemic was a particularly extreme shock to the system, but in a business as dynamic as retail, there is always a risk that forecasts based on how things used to be will fail to accurately capture how things are now or how they will be in the future. No demand planning solution, no matter how advanced, can ever completely escape forecasting errors.
That’s why it’s so important that the experts on retail demand planning teams are able to fully understand forecast errors. If their system provides transparency into how it forms the forecast, retail experts are able to quickly understand and correct any errors they see in it.
Too many retailers rely on “black box” forecasting systems that can take in all sorts of data to produce accurate forecasts, but lack transparency. Black box systems may well kill your business—or least your planning efficiency—for a couple of reasons. First, occasional extreme forecast errors can inflict far more damage on performance than smaller, more frequent errors. Second, when demand planners can’t make sense of forecast errors, it erodes their confidence in all forecast calculations, leading to increased double-checking and manual forecasting and undercutting the entire goal of harnessing computer power to automate your forecasting.
That’s why best practice retailers understand the value of transparency. Even when the system does the heavy lifting, human planners must always be able to both understand and control how their forecasts are generated.
4. Refine Grocery Replenishment for Improved Availability, Waste, and Efficiency
The quality of a grocery retailer’s store replenishment process has a direct impact on its top line revenue and bottom line profits.
High quality, forecast-driven grocery store replenishment consistently translates into the following benefits:
- Increased revenue from better on-shelf availability boosting sales by up to several percentUp to 40% lower markdown and spoilage costs as supply more accurately matches demandOptimized inventory flows enabling up to 30% reduction in goods handling costs in distribution centers and storesMuch more efficient capacity utilization in transportation, storage, and manual work phases throughout the supply chain
Yet, in a 2022 survey of North American grocery retailers, only 24% of respondents had implemented some level of forecast-driven store replenishment, and only 7% had implemented it extensively. Store replenishment is definitely an area where many supermarkets’, discounters’, and convenience stores’ operations are currently quite far from best practice.
4.1. Fresh Food Replenishment Requires Detailed Planning and Execution
For fresh products, well-managed store replenishment is central to finding the optimal balance between the risk of lost sales margins caused by stock-outs and the risk of waste or markdowns eating already slim margins.
Even though traditional supermarkets have decades of experience dealing with fresh products, many still do not excel in this area. Their supply chains are reactive enough to support frequent deliveries, but their replenishment planning is not up to scratch.
According to the North American grocers surveyed, the annual value of spoilage was on average more than $70 million, and up to several hundred million dollars annually for the largest companies offering a wide range of fresh products. A reduction of 10-40% would translate into somewhere between $7–28 million in annual savings. This is not only feasible, it is something that modern food retailers are expected to do in order to reduce their carbon footprints and make their businesses more sustainable. (Read more about reducing carbon footprints in supermarkets, convenience, food service, and e-grocery here).
4.1.1. Balancing Waste and Lost Sales
For so called ultra-fresh products, meaning short shelf life items that need to be sold that same day, 100% on-shelf availability means that there will always be waste or markdowns unless the forecast is consistently flawless on the day, store, and product level. This means that very granular control is needed to find the optimal balance between the risk of stock-outs and the risk of waste. Other fresh products face a similar challenge, just a bit less pronounced.
Demand for a product in a specific store typically varies between different weekdays. For some stores and products, this weekday variation in fresh replenishment can be very dramatic. This means that the same safety stock does not fit all weekdays when dealing with short shelf life products.
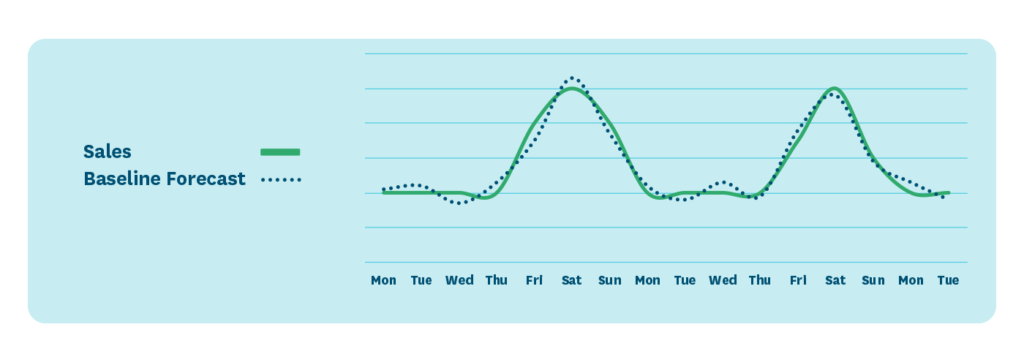
Roast beef, for example, tends to sell a lot more leading into the weekend than coming out of it. For roast beef – even when the day-level forecast is accurate – a static safety stock level leads to 1) excess inventory after the weekend, with increased risk of waste, and 2) perilously low safety stocks during the weekend, with an increased risk of stock outs.
To find the right balance between the risk for waste and the risk for stock-outs, safety stocks need to move up and down in step with the expected sales volumes and forecast errors for the different weekdays. Good retail planning systems do this kind of granular safety stock optimization automatically.
In fact, the best retail planning systems take optimization even further by not only enabling dynamic safety stocks, but optimizing each order based on cost-benefit calculations that balance the risk of waste against the risk of out-of-stocks. Such machine learning algorithms minimize the total of lost sales margin and cost of waste.
The cost function needs to be adjustable in terms of how much weight it places on on-shelf availability vs. waste to allow for considering the strategic roles of key categories and items as well as whether there are many or limited opportunities for substitution within the product category.

Figure 14: Best-in-class retail planning systems optimize each order based on cost-benefit calculations that balance the risk of waste against the risk of out-of-stocks.
When managing store replenishment of fresh products, it is very important that all calculations and optimizations are done automatically. It is an impossible task for any human to keep track of all factors influencing demand, such as weekday variation (e.g. seasons, weather, and promotions) as well as all factors influencing replenishment (e.g. delivery schedules, batch sizes and day-level probabilities of waste and stock-outs) for hundreds or thousands of items per day in a store, let alone hundreds of stores.
However, it is equally important that the forecasting and replenishment system does not turn into a black box. Actionable analytics allow supply planners to easily detect and remedy exceptions such as historical or projected waste or poor availability.
Examples of typical exceptions in fresh food replenishment are:
- Too large order batches causing waste in stores. Sometimes order batches, such as case packs, are so large in relation to a store’s demand that each delivery of the product will result in waste. To
address this problem effectively, your supply planners need to be able to determine whether this is a problem in just a few or many stores, what the financial implications are, and whether the problem can be mitigated by directing replenishment to specific weekdays, such as ordering the products only for the weekend.Too much allocated shelf space causing waste in the stores. Sometimes visual minimums, designed to keep displays attractively stocked, drive excess stock
and waste of fresh products. Supply planners must be able to identify whether the problem is isolated to a few low-demand stores or whether there is a more widespread problem with the planograms in use.Systematically poor availability or high waste on specific weekdays. Systematic patterns of poor performance on certain weekdays, such as higher than average waste on Mondays, is not uncommon. To address this problem, your supply planners need to understand the root cause
of this problem. There can, for example, be process issues, such as store personnel checking sell-by dates and recording waste on specific weekdays, which need to be accounted for in replenishment planning.
Automation radically reduces the time spent on routine tasks in store replenishment planning. At the same time, it multiplies the impact of your most knowledgeable process experts. If store replenishment has not been automated, your best supply chain analysts have limited leverage. They can review successes and failures in the rearview mirror and try to turn a few of their findings into action in the stores with help from the field training team.
When store replenishment is automated and replenishment planning centralized to a knowledgeable team, your planning experts can make a visible difference in hundreds of stores, almost immediately, simply by fine-tuning replenishment settings.
4.1.2. Stores Have Turned into Kitchens
With consumers increasingly looking for convenience, food-to-go and meal solutions are on the rise. Many stores are turning into kitchens where sandwiches, hotdogs, and salads are made.
Traditionally, products manufactured on-site have been considered special items that have to be managed manually in the stores. However, with the growing demand for ready meals, the importance of on-site production has grown much more pronounced and more critical to food retailers’ profitability.
The replenishment process of ready meals is not all that different from replenishing other products sold in a store. It is just a bit more complicated. The demand for the end products—the meals—needs to be translated into the ingredients used to manufacture the end products. The replenishment calculations need to be done for each ingredient accounting for each ingredient’s lead time and on-hand stock.
Essentially, the process is the following:
Forecast end product demand. Translate estimated demand for end products into estimated demand for ingredients needed to manufacture the end product. This requires knowing the recipe (sometimes also called the bill-of-materials, a term borrowed from the manufacturing industry) as well as the yields of the different ingredients. If 1.3 oz of lettuce is needed for a sandwich, the calculations may need to be done according to 1.7 oz of lettuce per sandwich to account for part of the lettuce not suitable for use in a sandwich.Calculate the estimated demand for each ingredient. The total demand for an ingredient often reflects its use in several end products.Calculate the required replenishment quantity of each ingredient based on lead time, available stock, potential incoming orders, estimated demand, and target safety stock.Sometimes, the ingredients included in a recipe are composed of other ingredients, such as special mayo or mustard produced on-site. In those cases, similar calculations need to be conducted for several levels of recipes. A horrible task for any human being, but quite manageable for a computer.

4.1.3. High-frequency Replenishment for Ultra-Fresh Products
For ultra-fresh products, many retailers have chosen to deliver them to stores multiple times per day to guarantee freshness. Similarly, items produced on-site are typically prepared in several batches during the day. This applies especially to the growing category of in-store bakery products, which ideally should still be warm when the customer picks them up. In addition, the new trend of food retailers opening small stores in urban locations has made several replenishments per day a must due to lack of in-store storage space.
Placing more orders per day or designing the optimal bake plan per day requires factoring in both weekday-related and within-the-day variation in demand. For some products, the within the day or so-called intraday demand pattern will follow the general customer footfall for that day; for other products, such as lunch items, demand is more influenced by how the items are planned to be consumed.
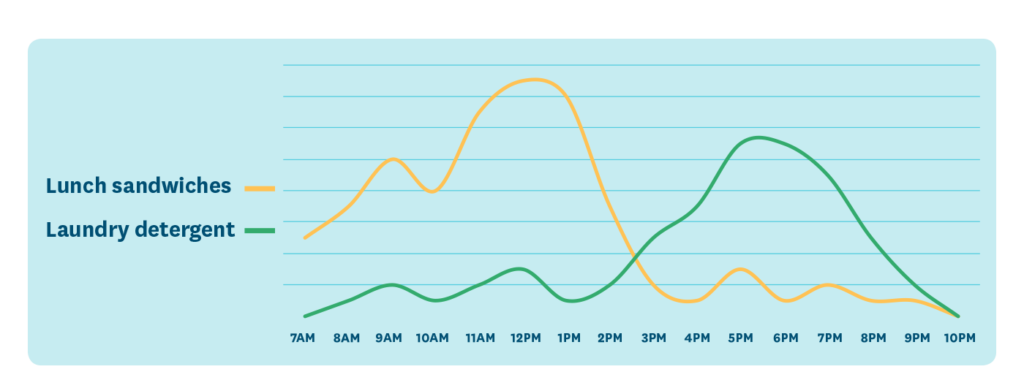
Again, keeping track of both weekday and intraday demand patterns manually is quite a complex and error-prone process. Yet, many retailers still rely on their store associates to figure this out on their own. This is a high-stakes gamble, as ultra-fresh products inevitably have a big impact on how consumers judge the quality of fresh products in a store.
Best-in-class retail planning systems can figure out the optimal split between multiple orders or production batches per day as well as adjust the quantities as needed, automatically.
4.1.4. Adding Science to the Art of Managing Fruits and Vegetables
Fruits and vegetables are often last in line when store ordering is automated. Obviously, produce faces the same challenges caused by short shelf life and variable demand as other fresh product categories. In addition, the varying supply and quality of fruits and vegetables demand additional flexibility from the planning system in use.
The regions from which fruits and vegetables are sourced constantly change as crops are harvested in different parts of the world different times. Even growers in the same region may have timed their crops slightly differently. Furthermore, as there is always some uncertainty in the availability of good quality product, food retailers usually try to ensure that they always have several vendors for the same product.
From a consumer perspective, a lemon is a lemon, but the supply chain may need to giảm giá with tens of different product codes for lemon, each associated with a different vendor. Effective management of fruits and vegetables requires the planning system to be able to seamlessly switch between planning levels as needed:
Demand forecasting needs to be conducted on the product level, for example “domestic organic tomato,” using historical sales data for all domestic organic tomatoes regardless of supplier. The replenishment quantity also has to be determined based on the available inventory of domestic organic tomatoes as well as their forecasted demand. The replenishment order, however, should be generated for the current vendor of domestic organic tomatoes. This is where the planning system needs to move from working on the product level to the SKU level, i.e. from “domestic organic tomato” to “domestic organic tomato supplied by GreenGrowers Co.” Often, the replenishment order needs to be split between two or three vendors to ensure availability in case of vendor product shortage as well as to keep several vendors in business. In that case, the planning system also needs to take care of allocating the order need to several vendors — for example 65% to GreenGrowers Co and 35% to OrganicFarmers Co.The forecasting and replenishment process for fruits and vegetables is highly laborious to manage manually but can be effectively automated. The key prerequisite is clear guidelines for which products are to be included in the stores’ assortments and which vendors are to be used for sourcing any given time. As in any automation process, high-quality master data is essential.
4.2. Optimized Center Store Replenishment Is Key to Supply Chain Efficiency
Fresh products need to be delivered to stores in perfect sync with demand. Center store products and other products with longer shelf-lives, on the other hand, offer more opportunities for an optimized flow of inventory in the supply chain. Optimized replenishment of center store products is key to lowering costs in stores and throughout the grocery supply chain.
Retailers that have mastered the replenishment of non-perishable products benefit from a much more level flow of goods through their distribution centers, enabling a much quicker return on investments in warehouse automation and reducing the risk of capacity bottlenecks having a negative impact on on-shelf availability. Furthermore, as grocery retailers’ store staff spend a lot of time and effort shelving products, optimized replenishment helps retailers to reduce operational costs in their stores.
4.2.1. Synching Replenishment and Shelf Space for Cost-Efficient Operations
Grocery retailers have traditionally operated in a very siloed manner with very little communication between the merchandizing teams responsible for store planograms, the supply chain teams responsible for store replenishment, and the store operations teams responsible for in-store work processes. This must change.
The space allocated to each product in a store has a big impact on both the results and costs of the store replenishment process:
- If the allocated space is very large in comparison to demand, the inventory needed for ensuring optimal on-shelf availability will not be sufficient for maintaining a visually appealing, full display. For that purpose, additional visual minimums need to be defined. Visual minimums
indicate how many units of a product need to be on the shelf to ensure that the display is visually appealing. For slow-sellers, the visual minimums will always be higher than the inventory levels required for great on-shelf availability. For long shelf life products this may not be a problem, but for fresh products, excessive visual minimums may cause unnecessary spoilage.If the allocated space is small in comparison to demand, incoming deliveries will not fit on the shelf. At least
part of the delivered quantity will need to be placed in a backroom or other storage area. This significantly increases the cost of shelf stacking, as goods need to be moved back and forth between the sales area and the backroom. In addition, the use of backroom storage significantly increases the risk of empty shelves, as timely replenishment from the backroom is dependent on the vigilance of store personnel.
Although surprisingly rare, full integration between space and replenishment planning is an important best practice for increased operational efficiency:
- Access to planogram data makes it easy to automate the maintenance of visual minimums on the product-store level based on the number of facings or total shelf space allocated to each product in each store. Access to planogram data makes it easy to automatically trim replenishment orders that would cause incoming deliveries not to fit on the shelf. Usually this
rule needs to be balanced with the risk of stock-outs if the space allocated to some products is very small in relation to their demand. Access to floor plan information enables assigning main replenishment days based on where products are displayed in the store, with the aim of creating more focused deliveries that minimize the need for store personnel to unnecessarily move around the store when stacking shelves. Access to planogram
information makes it possible to plan replenishment so that shelves are filled up to the maximum each time a delivery comes in, minimizing shelving work in stores. This means that rather than getting two batches in one go, if there is space for a third one that would be delivered next week, the order is calculated to fill the assigned shelf space upon arrival.
The space assigned to each product is of vital importance to how efficiently the replenishment process can function, so it is important to deliver continuous feedback to merchandizing. Good analytics tools will help you identify products and stores where there is a mismatch between space and sales, i.e. products and stores for which incoming deliveries do not fit directly on the shelves or products and stores where visual minimums lead to waste or markdowns.
Ideally, space planning should always be based on the detailed store-, product-, and day-level forecasts as well as information on replenishment cycles and main replenishment days available from replenishment planning:
- By using accurate forecasts rather than looking historical sales data when optimizing how space is allocated to products, the space planning team can more easily take seasonality and trends into account. Based on good forecasts of the expected maximum sales per delivery interval, shelf space can be optimized to be truly efficient
for all products in a store on all weekdays. This kind of optimization makes it possible to attain fewer deliveries and direct-to-shelf flows for a much bigger proportion of the product range.
We have seen forecast-based optimization of shelf space translate into up to 30% lower distribution and in-store replenishment costs.
4.2.2. Smart Replenishment For Efficient Store Operations And More Level Goods Flows
Typically, every large grocery retailer replenishes all or least most of its stores every day from its distribution centers. This is because fresh products demand frequent deliveries and because the overall inventory flows are substantial enough to warrant daily deliveries.
If all replenishment opportunities are used for all product groups without discretion, two problems will follow:
The deliveries to the stores will consist of a random mix of products from several product categories displayed in different parts of the store. This means that store personnel will spend a significant amount of time moving roll cages around the store to stock shelves (see Figure 17).The delivery volumes on different weekdays will not be roughly equal, but rather will reflect the daily variation in sales volume, often with significant delivery peaks towards the end of the week in anticipation of weekend demand. This leads to fluctuating capacity needs in both distribution centers and stores, which increases costs.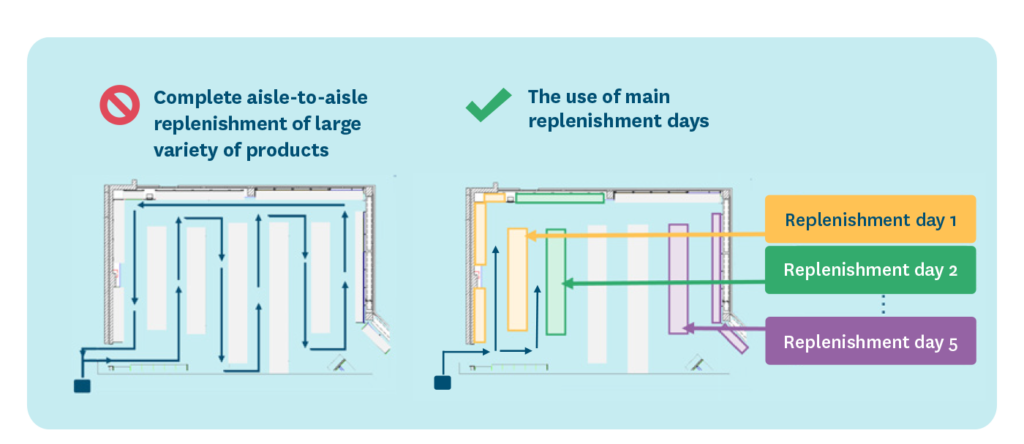
Instead of automatically using all available order or replenishment opportunities for all products, the best practice is to define main replenishment days for longer shelf life products. This means that replenishment of some center store product groups is concentrated to specific weekdays instead of being scattered throughout the week. Replenishment planning, such as the optimization of safety stock and calculation of order quantities, will be based on delivering the goods on the specified main replenishment days. However, to ensure the highest possible availability, replenishment orders are also triggered for the other available replenishment days to avoid stock-outs if there are unexpected demand peaks.
In practice, this means that instead of ordering detergents every day, fast moving detergents are primarily replenished on, for example, Mondays and Thursdays, and slow-moving detergents on Thursdays. For detergents, the other replenishment days from the distribution center to the store are only used in case there is a risk of stock-out in the store.
The use of main replenishment days allows for significantly more efficient in-store replenishment without hampering on-shelf availability. More consolidated deliveries make it more efficient for store personnel to replenish store shelves, especially when the main replenishment days are set based on what product categories are displayed in the same aisle or zone of a store. We have seen reductions of 20% in the time spent stacking shelves following the introduction of main replenishment days.
As with many other processes, the use of main replenishment days can be further optimized when the basics are in place. By using AI to optimize main replenishment days across the whole store, the inbound goods flow to a store can be leveled out across weekdays. In many stores, weekends can be very busy, with lots of customers doing their weekly shopping while large quantities of fresh products are being delivered to the stores. Setting main replenishment days for center store products to the quieter weekdays balances the incoming goods flow and makes personnel planning in the stores easier. (Read this case study for additional details.)
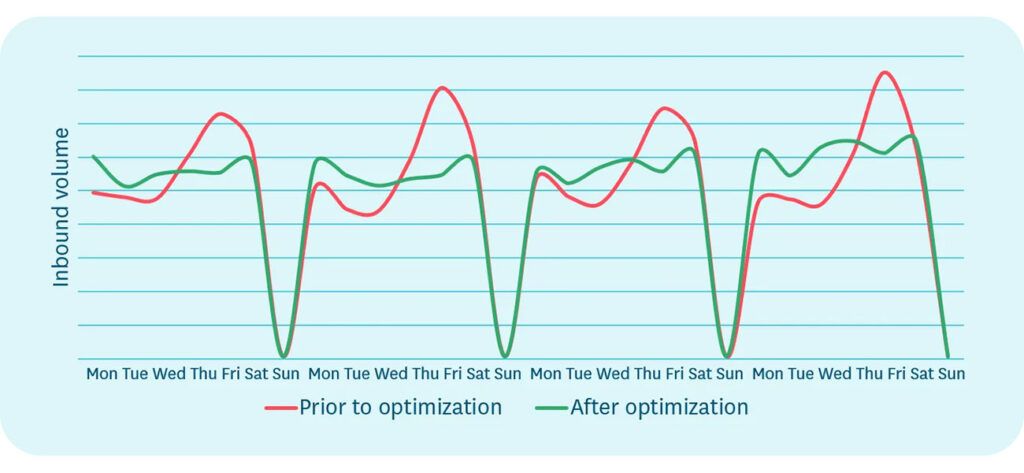
4.2.3. Dynamic Pack Sizes to Meet Dynamic Demand
One powerful tool to increase store replenishment efficiency is to optimize the use of different pack sizes. In many cases, stores can choose to order case packs, pallet layers, or full pallets from the distribution center. Larger batches are more efficient to handle both in the stores and the distribution centers, but clearly the deliveries need to match the available space and demand in the stores. Otherwise inventory will pile up in the stores and reduce rather than increase efficiency by congesting backrooms and causing multiple trips between the backroom and shop floor to replenish shelves.
Especially for retailers operating stores of different sizes, optimizing replenishment pack sizes per product and store has a direct impact on handling costs. However, doing it only once as a concerted effort does not suffice as demand changes over time and, for some products, also with the seasons. During the high season, a pallet might be most efficient while outside the peak, smaller case packs may be more efficient.
The retail planning system needs to be able to automatically optimize which pack size to use per product, store and order. This means that whenever an order is placed, the system always checks all available pack sizes—typically varying from the case pack to full pallets—and selects the most efficient pack size in relation to forecasted demand.
To attain the full efficiency gain, the supplying warehouses need to be able to estimate the demand for the different pack sizes. Otherwise they may end up in a situation where they use individual packs to put together pallets for the stores, rather than having full pallets flow through the distribution system. This is possible when the store projections (see Section 5) used as the basis for distribution planning reflect the stores’ forecasted use of different pack sizes.
5. An Integrated Supply Chain Driven by Customer Demand
Traditionally, store replenishment and inventory management the regional distribution centers or central warehouses have been separate processes, driven by separate demand forecasts.
In a 2022 survey, we found that 31% of large US grocery retailers still base their distribution center forecasts on historical data of outbound deliveries from these distribution centers. This is akin to driving a car while looking the rearview mirror.
According to the same survey, only 29% of respondents have chosen the more forward-looking approach of basing their distribution center forecasts on store demand forecasts. Granted, this is a better approach than only looking outbound deliveries.
There are, however, some important disadvantages to using store demand forecasts to drive planning the distribution centers:
Goods need to be delivered to the stores before the stores can sell them. This means that the distribution center forecast needs to go up before the stores’ demand forecasts go up and vice versa. The difference in timing depends on the stores’ sell-through rates and replenishment schedules, which means that the difference in timing varies between stores and products and sometimes also weekdays. The result is that it is almost impossible to accurately account for the difference in timing, which is bad news for your forecast accuracy the distribution centers.When goods are pushed rather than pulled through the supply chain, there will be outbound delivery peaks the distribution centers which are not visible in the stores’ demand forecasts. A typical example is promotions, where anything between 30% and 100% of the expected promotional uplift needs to be delivered to the stores before the promotion starts. The promotion, thus, causes a much bigger demand peak the distribution center than in the stores. This peak is fully controlled by the retailer itself, but still requires a lot of manual planning work or “guesstimation” when the supply planners the distribution center try to anticipate when and in which quantities stores will take in the promoted products.It is quite ironic that many of the situations considered most difficult to tackle in the distribution centers, such as building up stock in stores for promotions or new product introductions, are situations fully in the hands of the retailers themselves.
The best practice is to base distribution center forecasting on the stores’ projected orders, which reflect both pull-based demand as well as planned, push-based stock movements. In the 2022 survey, 40% of responding North American grocery retailers had implemented this.
To achieve seamless integration of store and distribution planning, the planning system needs to be able to calculate projected store orders per product, store, and day several months or even a year into the future, reflecting current and known future replenishment parameters as well as the demand forecast. These calculations, of course, require significant data processing capacity, which is likely to be one explanation for the surprisingly low adoption rates.
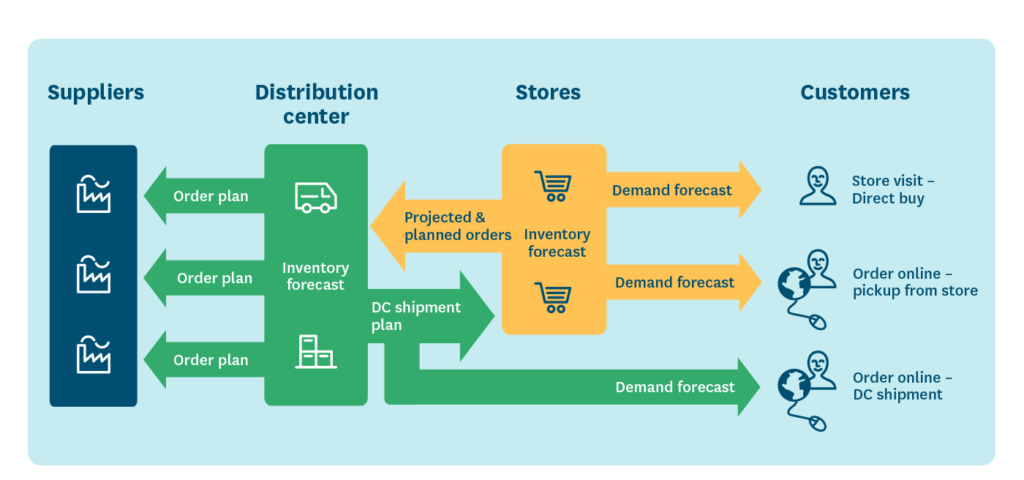
In practice, the stores’ order projections consolidate data on their current inventory, safety stocks and visual minimums, delivery schedules (including main replenishment days), as well as any planned inventory movements, including everything from stocking up to build promotional displays to shifting orders to level out the capacity requirements in distribution.
Table 1 presents some examples of situations in which the value of basing forecasting the distribution centers on projected store orders is especially notable.
Product introductions When launching a new product, least one case pack or a sufficient amount of product to fill up the allocated shelf space is pushed out to each store. This creates inventory buffers in the stores, which will take days or weeks to digest. As long as there is surplus inventory in the stores, the projected store orders (as well as the actual outflow from the distribution centers) will be lower than forecasted consumer demand. Product terminations When a product termination has been planned in advance, the distribution center forecast will automatically go down as the termination date draws closer, supporting a controlled ramp-down of inventory. When the distribution center forecast is based on projected store orders, the forecast automatically considers the existing inventory buffers in the stores and accurately estimates how long it will take to clear out the remaining stock in each store. Promotions Typically, anywhere between 30 – 100% of the expected promotional uplift is pushed to the stores in advance of a promotion. The good news is that these planned inventory movements are completely predictable (as they are in fact planned, with no need for forecasting) and will automatically be included in the projected store orders. Also, if the stores are left under- or overstocked following the promotion, the stores’ fulfilment needs will be accurately reflected in the distribution center forecasts. Seasons Almost always, some buffer stock is distributed to the stores before the start of a major season. This can be due to the need to create nice seasonal displays in the stores, level out seasonal peak volumes, or due to the season being weather-driven making the exact timing of the season start somewhat uncertain. As with promotions, these planned stock movements will be automatically visible in the stores’ projected orders used as the forecast for the distribution centers. Furthermore, as seasonal demand may vary a lot between stores, for example due to local weather conditions, the stores’ inventory buffers will be consumed different paces. This will be automatically visible in the forecast for the distribution center. Changes in replenishment schedules It is not uncommon that stores’ replenishment schedules are changed either temporarily, for example to match increased demand in the high season, or permanently, for example following the implementation of new transportation routes. Changes in the replenishment schedule will, naturally, not have an impact on consumer demand but they will have a direct impact on the goods flow into the stores. The resulting changes in the timing and size of the deliveries to the stores will automatically be captured in the distribution center forecast when it is based on projected store orders.Table 1: Examples of situations where the use of projected store orders, rather than stores’ demand forecasts, allows for much more accurate planning the distribution centers.
When the order projections are aggregated across all stores, they form a very accurate, customer-driven forecast for the distribution centers.
Additional benefits of the supply chain integration include supply chain transparency supporting capacity planning, supplier collaboration (discussed in Section 7) as well as straightforward handling of cross-docking, pick-to-zero, and shortage situations.
5.1. Plan Once and Execute Automatically Throughout the Supply Chain
When planning the distribution centers is based on the stores’ projected orders, the impact of planned activities, such as promotions or pre-season allocations, are immediately visible throughout the entire supply chain. To reap the full benefits of this transparency, all planning data needs to be made available to the planning system as soon as a promotion plan, assortment change, price change, or any other relevant decision has been made.
A planning system that supports time-dependent master data is a key enabler of proactive planning. Below are just a few examples of how time-dependent master data enables you to register valuable information immediately when it becomes available. This, in turn, allows your replenishment planners to rely on the planning system to automatically trigger the necessary actions the right time with very little manual work.
- Time-dependent replenishment schedules: When store replenishment schedules can be managed using dates, it becomes possible to update the planned future replenishment schedules into your planning system as soon as the information becomes available. This enables replenishment planners to
trust the planning system to automatically consider these changes both in replenishment planning and when calculating supply chain projections.Assortment activation and termination dates: When start and end dates for the active product range have been defined, product ramp-ups and ramp-downs are much easier to manage. Routine planning tasks, such as pipeline fills for new products or inventory ramp-downs for products to be discontinued, can be automated. This
automation reduces manual work, but also ensures optimal inventory levels in all phases of a product’s lifecycle.Stock-ups before promotions: Promotions naturally have start and end dates, but it is equally important to be able to specify beforehand how stores should be stocked. It is usually ideal to define how many days before the promotion the promotional goods should arrive in the stores, what stock quantities stores should receive to be able to build the
planned promotional displays, and what proportion of the forecasted promotional demand the first deliveries should cover. Rules and templates make it possible to attain accurate replenishment plans for each store and product without manual work.Temporary supplier delivery restrictions: Suppliers may have temporary delivery restrictions. Chinese manufacturers may, for example, not dispatch shipments during the Chinese New Year. If information like this is made
available to the planning system, the system knows to put in orders for this period early enough to ensure high availability during the affected period, while minimizing manual work and dependence on human memory.
An integrated supply chain set-up removes the need for double-planning work. The impacts of planned changes in store replenishment are automatically reflected in the projected store orders forming the demand forecast for the distribution centers. This means that as soon as the required store stock-ups for promotions are planned, they will be visible in the distribution center forecast on the right dates and in the right quantities.
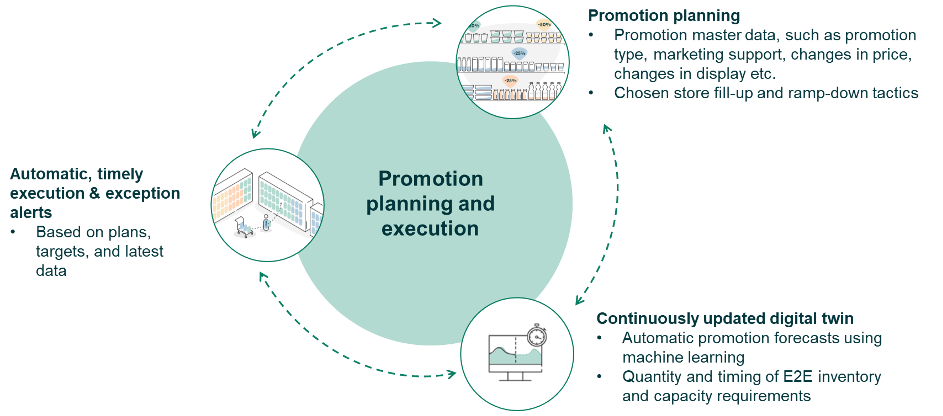
Of course, having the right functionality in your planning system is a key enabler, but the real challenge is getting the whole organization to work more proactively. Ensuring that decisions are made early enough, but not too early to unnecessarily reduce flexibility in a dynamic market, requires that everyone in the organization has a basic understanding of how the supply chain works and what the relevant lead times for different types of decisions are.
5.2. Multi-echelon Optimization of Goods Flows
An integrated supply chain makes it possible to manage multi-echelon inventory flows efficiently, with minimum waste and a high level of automation. When all data on demand forecasts, available stock, delivery schedules, lead times and batch sizes for all supply chain echelons is available in the same planning system, it enables seamless optimization of inventory flows throughout the supply chain.
Cross-docking is an inventory strategy aimed maximizing transportation efficiency while minimizing handling costs. Cross-docking is often applied to bulky products, such as drinks, to attain lower storage and handling costs. It can also be used to cut lead-times for short shelf life products. In a cross-docking set-up, goods are delivered from the supplier to a cross-docking facility where the goods are put not into storage, but moved from the inbound truck to an outbound truck for distribution to stores.
There are some requirements for cross-docking to work efficiently: 1) Suppliers need to be able to deliver full truckloads to the cross-docking facilities, 2) the delivery units, such as pallets or roll-cages, need to be ready for immediate movement to the outbound trucks without additional handling, and 3) the outbound trucks need to get a high fill-rate to keep transportation costs down. The planning system, thus, needs to optimize both inbound and outbound flows to and from the cross-docking facilities as well as understand the total lead time from supplier to store.
Another example of an inventory policy that requires integrated supply chain planning is pick-to-zero. In this inventory strategy, orders to the suppliers are based on the stores’ replenishment needs. However, rather than fixing the quantities to be delivered to each store, the supplier delivery is reallocated to the stores upon receipt based on the latest inventory and forecast information. This allows for adjusting the delivery quantities per store in case the supplier could not deliver in full or in response to potential unexpected demand peaks in the stores after the original replenishment need was calculated. As a result, supply matches demand more accurately than when using the traditional cross-docking approach. The pick-to-zero approach can be seen as a way of shortening the order-to-delivery lead times to the stores, as the store-specific quantities are finalized not when ordering from the suppliers but when preparing the goods for store distribution.
When supply chain planning is fully integrated, exceptions can be resolved in an optimal and automated fashion. Let’s look inventory scarcity due to, for example, an incoming shipment being delayed. Instead of fulfilling store orders on a first-come, first-served basis, the available inventory can be automatically allocated to stores to maximize overall on-shelf availability or in accordance with a tactical prioritization of the stores. In the best case, on-shelf availability is not even affected. In a similar manner, inventory batches nearing their expiration dates can proactively be forced out to the stores that have the best chance of selling the products full price.
6. Efficient Inventory Management in Distribution Centers
Replenishment of central warehouses and distribution centers is sometimes seen as more of an art than a science. It is true that longer lead times, especially when ordering overseas, and lack of control over external suppliers introduce complexities. Yet, least in principle, replenishing central warehouses or distribution centers is not that different from replenishing stores.
When replenishing stores from their own distribution centers, retailers can optimize order fulfillment as they find best. When ordering goods from suppliers, though, there may be complex restrictions regarding minimum order value or quantity. In addition, there may be volume-based discounts or other rebates which, when efficiently exploited, can have a significant impact on margins. Many retailers have not been able to put this kind of supplier contract or price information into their planning systems, making it necessary for the operative buyers to invest significant time double checking orders.
When replenishing stores, the active goods flows (combinations of products and stores) for any larger retailer is typically measured in millions or tens of millions, which means that automation is crucial. For central and regional warehouses, the number of order lines is much smaller and the value per order line much higher, making the economic impact of each order line more pronounced. This has both enabled and encouraged a lower degree of automation in operative buying compared to store replenishment.
We have found that setting up operative buying processes in a structured way with good system support can also result in very high levels of automation the distribution centers. This does not, however, necessarily mean that best practice retailers have a significantly leaner buying team. A key result of increasing the automation of routine tasks is that operative buyers have more time to proactively giảm giá with potential capacity, delivery or quality issues and to analyze the performance of the current assortment, suppliers and supplier agreements for continuous improvements.
6.1. Total Cost Optimization of Inbound Flows
As the inbound goods flows to distribution centers are more consolidated than the outbound flows, there are more opportunities for order optimization when replenishing distribution centers than when replenishing stores.
It is important that the planning system can perform order optimization on multiple levels to reach the most cost-efficient outcome.
Some examples of order optimization on different levels are:
- Calculation of the economic order quantity (EOQ) per product to minimize inventory and handling costsSelecting the optimal order batch
size—such as case pack, pallet layer or full pallet—when several order batch options are available, considering potential price differences between the different batch optionsBuilding mixed pallets for efficient transportation and goods handlingBuilding orders that fill one or multiple load carriers, such as trucks or containers, or that meet suppliers’ order restrictions, such as minimum order value or minimum number of pallets
Although it seems simple, the process of pooling orders for multiple products to fill load carriers or meet supplier order limits can be quite the test for your planning system’s flexibility.
To meet supplier requirements and benefit from lower transportation costs or supplier discounts without accumulating excess stock, you typically need to be able to:
- Flexibly define which products should be pooled when planning an order. Products from the same supplier are often pooled together, but sometimes
the same supplier’s different manufacturing sites should be considered separately or all products sourced from the same region, regardless of supplier, seen as one group.Set targets and/or limits for the consolidated order in multiple units, such as value, volume, number of pallets, weight or combinations of these dimensions. For example, when filling trucks, you want the order to fill the available space efficiently so as not to incur costs for transporting air, while the same time
ensuring that the legal maximum weight is not exceeded.Let the planning system decide which kind of a load carrier it should aim to fill up with the order. With some suppliers, it may make sense to sometimes order a truck, sometimes order a truck and a trailer, and sometimes two trucks, only one of which has a trailer, depending on the forecasted demand.Set the right order trigger level. When supplier order restrictions are difficult to meet, it may make sense to require enough
demand for, say, least 30% of a truckload before the planning system starts building an order that fills up the entire load carrier.
In addition to letting the planning system do the heavy lifting when it comes to supplier order requirements, the best practice is to constantly evaluate these restrictions and their impact on the flow of goods. Multi-year contracts in a dynamic market or fixed order restrictions for products with seasonal demand may turn out to be costly or infeasible as demand changes.
To support this, the ideal planning system should highlight all order suggestions more/less than needed as a result of these constraints, as well as show the difference from the actual need. Furthermore, it should provide analytical support to help the operative buyers make rational decisions concerning whether the benefit, such as rebate, of meeting a supplier restriction is greater than the resulting increase in inventory carrying cost and risk of obsolescence.
6.2. Smart Buying Takes Advantage of Good Prices
Retail costs are dominated by the cost of goods sold. The operative buying team needs to take responsibility for efficiently exploiting rebates to improve gross margins.
In theory, smart buying when prices are changing is quite straightforward:
- When you know that the price of a product will go up, stock up just before the price increase.When you know the price
of a product will go down, only order the quantity you absolutely need before the price change, and then stock up after the new price has come into effect.When a price will be lowered temporarily, for example due to a supplier chiến dịch, order less just before the price reduction and stock up when the price is low.
To be able to truly benefit from price changes, you also need to factor in your inventory carrying cost, time your orders correctly relative to when the price is changing, and potentially split the investment buy—the additional quantity you are buying above what you would need to meet demand—into several orders.
To further complicate things, there may be other factors that have an impact on the optimal order quantity. For fresh products, shelf life is always a factor. It makes absolutely no sense to stockpile inventory that will end up as waste, or to harm your reputation by putting goods with unattractive expiration dates in stores. Furthermore, in situations where storage space is scarce, the cost of inventory may suddenly jump to a whole new level if you exceed the capacity limits of your current warehouses. When your storage space is very full, you would need to find additional space outside of your current warehouses for additional goods, quickly turning your investment buy into a very unprofitable move.
The best practice is to feed your planning system with time-dependent price data to let the system optimize when and in what quantities to buy when prices are changing. In this way, you can take advantage of even minor price changes effectively, as the operative buyers do not need to spend time manually figuring out the optimal order quantities. It is important to keep in mind that restrictions, such as shelf life for perishable items or capacity limits on storage space, need to be considered. If your planning system is not able to giảm giá with such restrictions automatically, the suggested investment buys will need to be double checked by the buying team.
It is not unusual to have supplier contracts include a rebate triggered by the buyer’s annual order value exceeding a set quota. Again, keeping track of supplier quotas, placed orders, and forecasted orders is very hard to do manually. Intelligent planning systems support smart buying decisions by suggesting additional orders to get the rebate when feasible and by not suggesting any additional orders that would result in counterproductive stock-piling.
6.3. Batch-Level Inventory Management of Perishables
It is currently impossible to know the exact expiration dates of on-hand inventory in stores. It can even be hard to get a decent estimate if there are several batches of a product simultaneously on the shop floor, as some consumers work hard to find the freshest products available.
However, distribution centers are a different story. In distribution centers, modern warehouse management systems ensure that inventory is shipped on a first-in-first-out basis. In addition, they keep track of the exact expiration date for each batch in stock.
Making good use of batch-level expiration data in inventory management reduces waste and improves your service level:
- When the planning system is able to calculate projected spoilage based on forecasted demand and the expiration dates of the available inventory, it can order a sufficient
amount of goods to replace soon-to-expire inventory before the products expire. This significantly improves the service level of your distribution centers towards your stores.Best-in-class planning systems trigger early warnings when there is a risk of waste. This enables efficient force-outs to those stores most likely to be able to sell the products a good price, or it gives you time to find other sales channels, such as discounters, which may be willing to take the batches off
your hands a discount.The spoilage projections also show if there is a systematic risk of waste, for example due to products having large order batches compared to demand.

6.4. Real-time Data for Buying Fresh Products
For perishable products, a very high inventory turnover both in stores and in the supplying distribution centers is a must. This means that the supply chain is very vulnerable to quality issues, delivery problems or unexpected peaks in demand. In situations where store requirements exceed available inventory, quick reactions are of essence.
In many cases, suppliers of short shelf life perishables make several daily deliveries to the same distribution centers. This is partly to guarantee freshness and partly to level out volumes.
Several daily supplier deliveries make it possible for a retailer to accommodate actual demand by placing the orders as close to the different ordering deadlines as possible, making use of the latest demand and inventory data.
However, to be able to identify demand surges, the planning system needs to be tightly coupled with the underlying transaction systems and have access to real-time data. Of course, the planning system also needs to be able to process the data quickly enough to turn the latest data into orders as accurately as possible.
Similar quick reactions and within-the-day calculations based on real-time data are valuable when fruits and vegetables, which are prone to supply and quality issues, are received in the morning. Because the actual available inventory may differ from what is planned, it makes sense to re-allocate stock based on the latest forecast and stock data from the stores rather than fulfilling store orders in an arbitrary order.
7. Planning for Optimal Capacity and Resource Utilization Throughout the Grocery Supply Chain
In a dynamic business like retail, capacity bottlenecks can emerge in almost any part of the supply chain in response to a range of events, from holidays or unusual weather to promotions or big assortment updates in stores.
To identify and proactively resolve these bottlenecks, retailers need to understand how the forecasted demand will impact inventory, capacity, and resource requirements throughout their supply chains.
7.1. Retail Sales & Operations Execution (S&OE)
The S&OE process aims to ensure that retailers can fulfill short-term demand for the upcoming 0–3 months as cost-effectively as possible. The starting point is a very granular demand forecast the SKU-channel-day level (see Section 3.1). From there, planners can use supply chain projections (see Section 5) to get a detailed understanding of inventory, capacity, and resource requirements throughout the supply chain.
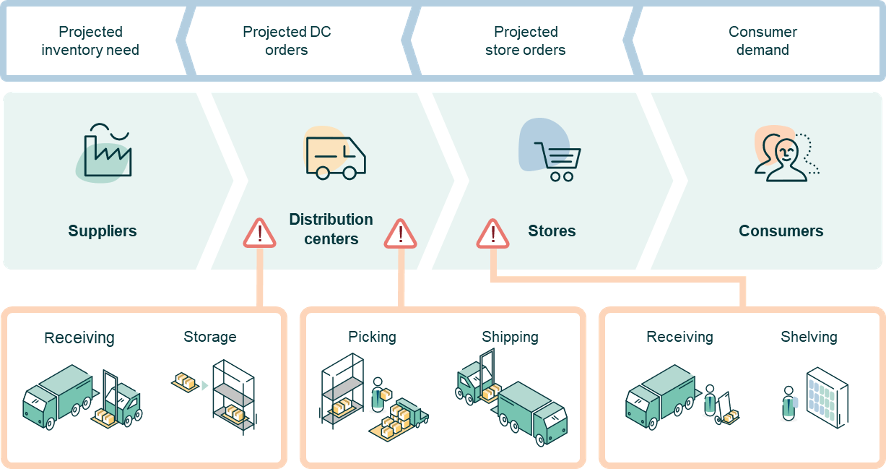
This end-to-end visibility into retail operations drives many S&OE benefits, including:
- Cross-functional alignment: Visibility makes the impact of business decisions made in one function instantly available
across all functions to be accounted for in their planning. For example, the expected impact of a planned promotion is immediately reflected in all local demand forecasts as well as inventory and resource projections throughout the supply chain. This means that business decisions like
promotions only need to be planned once for automatic execution.Proactive exception management: With visibility into current and future inventory, capacity, and resource requirements throughout the
supply chain, the system can automatically detect potential bottlenecks and help planners either prevent or quickly resolve them. For example, if the system alerts planners that an upcoming promotional stock-up combined with seasonal allocations will create an exceptionally large delivery peak, they can proactively manage the problem before it starts congesting store backrooms or
exhausting DC picking capacity. Effective contingency planning: With digital twin modeling, retailers can compare and better understand how different planning scenarios will impact their supply chain. For example, if a sales region is outgrowing its fulfillment capacity, a distribution planning team can easily model when and how to shift
fulfillment for some of this regional demand to another fulfillment center, effectively balancing capacity requirements across the distribution network.
When dealing with millions of goods flows, tens of thousands of employees, hundreds of vendors, frequent promotions, and regular price and assortment changes, there are bound to be exceptions to any retailer’s plans. Many of these exceptions require immediate attention and quick adjustments to avoid or minimize any negative impact on S&OE.
However, by turning to AI and advanced optimization, retailers can actually resolve most of these deviations without any human intervention. Best-in-class, autonomous issue resolution increases the speed and accuracy with which a retailer can manage exceptions by:
- Autonomously rebalancing inventory in the supply chain: triggering optimized scarcity allocations
and force-outs in line with the retailer’s business priorities, for example, or re-optimizing store orders on the fly in accordance with a pick-to-zero fulfillment approach. Ensuring throughput and efficient use of capacity: proactively
smoothing out fluctuations in goods flows (see Figure 23), continuously optimizing replenishment and space
to reduce in-store goods handling, and
optimizing workforce deployment using continuously updated workload forecasts.Recommending corrective or “next best” actions: automatically suggesting optimal markdowns to clear excess stock from
the supply chain, for example.
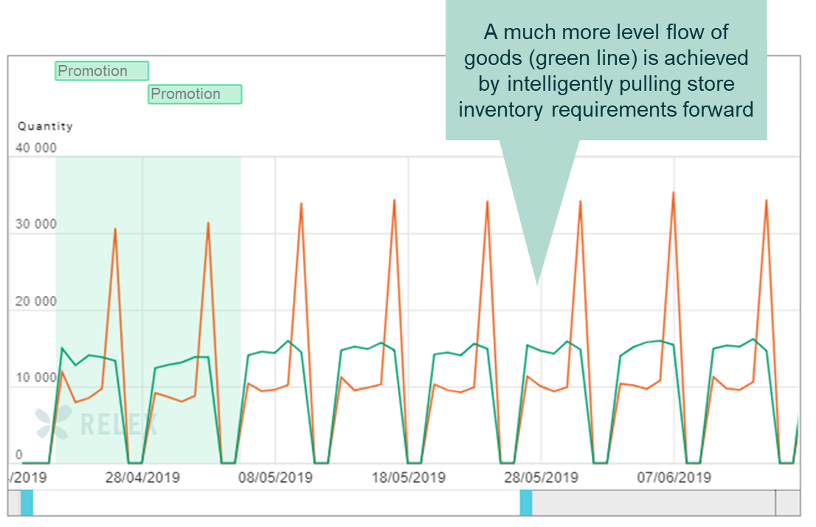
The best-performing retailers are able to combine human expertise with technology, quickly adapting to new situations and implementing new business priorities scale. A good case example of this is German drug store retailer Rossmann, who took only two days to stand up entirely new planning configurations that prioritized the delivery of essential products to stores during 2022’s COVID-impacted demand shift.
7.2. Retail Sales & Operations Planning (S&OP)
If the goal of retail S&OE is to resolve unforeseen capacity and resource challenges in the short-term, then retail S&OP, by contrast, looks further into the future. The goal of retail S&OP is to ensure sufficient capacity and resources to support future growth targets, planned changes in the distribution network, major seasons, and more.
Preparing for major holidays provides an important use case for retail S&OP, with the winter holiday season being the most important (and challenging) in most markets. Holiday seasons are generally marked by higher-than-normal demand that increases steeply until the holiday peak. After that peak, demand usually drops back to normal—or even dips below normal levels for a while.
But the retail S&OP process is about more than supply chain efficiency alone—it’s about maximizing profitability. S&OP should result in:
Goods flows that match, rather than exceed, capacity throughout the supply chain. This secures reliable supply, which in turn prevents lost sales from delivery problems.Cost-effective operations, with costly overtime minimized in all parts of the supply chain.Informed decisions that still support company profitability when capacity restrictions prevent guaranteed availability for all products.Full transparency into resource requirements, making it possible to ensure that all resources, including store workforce availability, are based on the same operational plan.Retail preparations for a major holiday like Christmas usually start around six months before the season begins. The first step is to agree on the constraints: will there be changes in delivery schedules/lead times or supplier capacity constraints due to the holiday season?
After agreeing to the constraints, the next step is reviewing sales and delivery plans to identify potential bottlenecks, which can emerge anywhere in the supply chain throughout the holiday season. Potential bottlenecks might include overly large deliveries adding to store congestion on busy days; days when warehouse staff face more order lines than they can pick; days when a warehouse receives more frozen products than it can store; and so on.
Using spreadsheets, it would simply be impossible model the supply chain—with all its complexities—accurately enough to proactively identify bottlenecks. Even building a simplified model would be enormously time-consuming and prone to error. The only way to detect the types of moving capacity bottlenecks described above with any degree of certainty is to use supply chain projections.
Once they’ve identified potential bottlenecks, retailers should use “what-if” scenario planning to examine and eliminate them. Bottom-up scenario planning allows retailers to see exactly how changes in delivery timing, replenishment schedules, or forecasted sales volumes would impact goods flow.
Typically, food retailers need to deliver long shelf-life products to stores earlier to không lấy phí up capacity to effectively manage fresh products in their high season. Different strategies and scenarios for leveling out the goods flow ahead of a holiday season include:
- Filling up store shelves: For many center store products, full shelves could cover demand for many weeks. Since waste is not an issue, it makes sense for retailers preparing
for the Christmas holiday to fill those shelves early, in November, so fresher products can be delivered in December.Allocating longer shelf-life products to stores: Fill-ups respect the assigned shelf space in the stores, but that isn’t always enough. Sometimes, it makes sense to allocate the demand for the next 2-4 weeks to stores in one go. Stores can house those items in a backroom and replenish from there as needed. While not suitable for all products, this
approach can be useful for special cases.Adjusting delivery schedules: Most food retailers make frequent store deliveries regularly, even outside of the holiday season, but if needed, it may make sense to schedule additional deliveries to help meet the increased demand.
Retailers should use their software’s scenario planning capability to identifiy the scenario that best meets their goals and come to an agreement with their suppliers on it. This enables them to lock their plan well in advance of the season, so they can then focus on execution and corrective actions.
An effective S&OP process leads to more level capacity utilization throughout the season. Furthermore, planners know of and can plan for any remaining peaks remain beforehand, rather than scrambling to manage costly surprises as they pop up.

7.3. Efficient Supplier Collaboration
Supplier collaboration has been a point of discussion for decades, but surprisingly few retailers have implemented it extensively. To establish fruitful collaboration, both parties need to both put in effort and receive measurable benefits from the process. Unfortunately, because this has rarely been the case, many collaboration initiatives fail.
While technology doesn’t solve the challenge of supplier collaboration, it can ease the pain. For example, most collaboration projects spend the majority of their effort just on collecting data from various sources, but the right planning system can minimize that work. Rather than trying to fix everything in one go, we recommend building your supplier collaboration processes bit by bit.
A good starting point is sharing order forecasts with your suppliers because it’s a lean way to collaborate. If your planning system can calculate supply chain projections, then the purchase order forecast, which tells your supplier what you plan to buy from them in the weeks and months to come, is already readily available to you. A good system can send automated reports that share that information with your suppliers.
You can also share relevant information on planned promotions, upcoming events, or other changes, helping your suppliers understand the reasoning behind your purchase order forecast. Retailers may also share demand forecasts or point-of-sale (POS) data with their suppliers, but the most essential information is what you expect the supplier to deliver and when.
A more collaborative way of working requires both parties to recognize the value that investing their time and effort will bring. Whereas simply sharing a forecast is one-way communication, Collaborative Planning, Forecasting, and Replenishment (CPFR) is true two-way communication. A good planning system helps by providing reliable projections of future purchase orders, analytical tools to understand potential changes and issues, as well as a platform or portal for the collaboration.
Ideally, suppliers can simply be given access to a retailer’s view of their projected demand, plans for purchase order placements, and data on promotions, seasons, events, etc., then add their own view. Combining the supplier’s holistic view into their categories and products with the retailer’s understanding of its business and marketing activities in this manner ultimately results in a more accurate overall plan.
Best-in-class planning systems can support this kind of collaboration by providing a platform that can take in multiple forecast types, alert users to any differences, allow users to edit plans, and finally, disaggregate the agreed plan to whatever level of detail is needed—whether stores, products, or days—to support operational execution.
8. Conclusion: Team up with the Machines to Win
Retail is in turmoil, and it is unclear what the impact of the different sales and delivery channels, store formats or even retailer players will be. In 10 – 15 years, we will probably look back this time in amazement and wonder “How did we not see this coming?”
Some predictions about the future of food retail are, however, easy to make:
Food retailers will waste less. It is a disgrace to spend so many resources growing, transporting and handling food products just to have them end up in the dumpster behind a supermarket. Grocery retailers must and will take responsibility for significant reductions in food waste, and, because waste eats profit, their efforts will also be great for their businesses.The food retail supply chain will become more effective. Consumers have become very price conscious and will not accept premium prices to keep inefficient supply chains in business. No one benefits from wildly fluctuating workloads or capacity requirements caused by poor planning and management, so neither retail employees nor management should be sad to see old, inefficient practices go.Technology and automation will play a big part in the transformation of retail. We have already seen this in other sectors that once relied heavily on manual routine work. There is no reason why retail would not go down the same path.To summarize, retail supply chains need to become more responsive and finely controlled than ever before to meet the demand for fresh products with minimum waste. At the same time, retail supply chains need to become more efficient by optimizing inventory flows from multiple perspectives—store operations, distribution, picking and warehousing—to meet the price pressure. This is only possible by teaming up with the intelligent machines.
The world of food retail is too complex to be managed with notepads and intuition. This has, of course, been true for a long time. The breaking news is that not only are the simplest jobs being automated, but significantly more advanced planner roles are being filled by machines, too. More importantly, intelligent automation will not only replace manual work, but take planning to a level of granularity never before seen.
Will there then be any role for humans in this brave new world? Yes, there will be plenty. Three important roles are:
Master of the machines: We are making great progress in specialized AI, the kind of machine intelligence useful for solving very specific tasks. However, we still need to have talented people designing the systems and determining when and how the available machine intelligence is best used.Colleague to the machines: Machine learning algorithms are very dependent on access to data. They have a hard time applying common sense or coming up with innovative solutions in new scenarios with insufficient data. This is where their human colleagues can provide invaluable insight.Innovators, thinking beyond the machines: Especially in businesses going through creative destruction, there is a great need for novel thinking, new business models and new ways of delivering food and services to consumers. Retail innovation is still far beyond the capabilities of AI.So please, do not hold your breath waiting for AI to revitalize your retail business or even solve your supply chain challenges. But please do start phasing in the use of machine intelligence where most feasible and impactful. This collection of best practices is a good place to start.